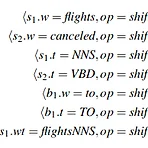
"해당 포스팅은 Dan jurafsky 교수의 Speech and Language Processing Chapter 14. Dependency Parsing 을 요약한 것이며 모든 이미지 자료로 책에서 인용한 것입니다" 해당 포스팅 전체에서 child node 가 단 한 개의 parent node 만 갖는다고 가정한다 1. Transition-based approach Transition-based 는 Parser (=predictor / oracle) 이 sequence of operator (left arc operator, right arc operator, shift operator, reduce operator) 를 predict 하는 방식. Each step 기준으로 보면 current ste..
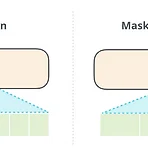
Transformer 구조라는 건 encoder - decoder 로 이루어진 seq to seq model 이고 거기에 attention mechanism 이 핵심 요소이다. BERT 는 transformer 의 encoder blocks 만 있는 모델 GPT2 는 transformer 의 decoder blocks 만 있는 모델 BART 는 bert encoder + gpt2 decoder 이라는 것. 그럼 결국 transformer 아닌가..? => architecture 이랑 pre-training 에서 조금씩 차이가 있다고 함 BERT 는 그니까 self attention + feed forward layer 로 이루어진 encoder block 의 stack 으로 이루져 있고 GPT2 는 ma..
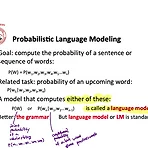
"해당 포스팅은 Dan Jurafsky 와 Chris Manning 교수의 2012년 Stanford NLP 강좌를 정리한 내용입니다." 석사논문 쓰면서 수없이 봤는데 누가 설명해보라고하면 자신 없을 것 같았던 N-gram language model 드디어 정리해본다. 우선 Probabilistc language model 이란 뭐냐? Sentence / phrase 가 주어졌을 때 그게 얼마나 likely 한지를 probability 로 나타내는 모델임. 그럼 그걸 어떻게 계산하냐? 2가지 방법이 있다. 첫번째로 Joint probabilty 인 $P(W)$ 즉, $P(w_{1}, w_{2}, w_{3} ...w_{n-1}, w_{n} )$ 을 구하거나 아니면 conditional probabilty ..
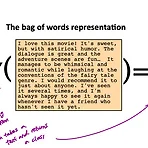
"해당 포스팅은 Dan Jurafsky 와 Chris Manning 교수의 2012년 Stanford NLP 강좌를 정리한 내용입니다." NLP 에서 Dan Jurafsky 만큼 설명 간결하고 알아듣기 쉽게 하는 사람 없을듯... 최고 그동안 나름 많은 NLP 강의를 들으면서 수도 없이 들었던 Naïve Bayes, Baysian Rules... 근데 용어 정리가 안 되다 보니 용어만 듣고서는 이게 뭐더라? 하는 경우가 너무 많아서 back to basics 을 통해 정리 할 필요성을 느꼈다. 그 첫번째 주제가 바로 Naïve Bayes! 바로 고고. 배우면서 등장한 용어는 핑크 형광색으로 표시해놨다. 1. Text Classifier Model 이란? 아래의 그림처럼 텍스트가 주어졌을 때, positi..
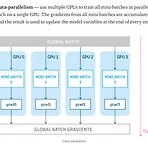
batch-size 는 중요한 hyper parameter 중에 하나임. Batch size 가 너무 클 때: overfitting Batch size 가 너무 작을 때: noisy / slow convergence => 하나의 data sample 미치는 영향이 너무 커지기 때문에 each step 이 noisy 이 때, GPU 가 하나밖에 없고, memory limitation 도 있어서 내가 원하는 batch-size 로 training 하기 어려울 때 쓰는 테크닉이 바로 Accumulated gradients ! GPU memory 가 부족할 때는 mini-batch 란 것을 또 사용할 수 있음. batch 1개당 error 가 back propagate 되면서 optimization step 한..
Traditional word embedding 방식은, fixed vocabulary 가 있고 각 vocabulary 마다 학습된 word embedding 이 있는 형태다. 마치 사전처럼 각 단어마다 그 단어의 word embedding 이 있어서 원하는 단어의 index 만 알면, 그 단어의 word embedding 을 가지고 올 수 있다. 반면, 이 단어의 word embedding 은 항상 고정된 상태로 (static) 바뀌지 않는다. 문제는 동음이의어의 단어를 embedding 할 때이다. Sentence 1: The mouse ran away, squeaking with fear. Sentence 2: Click the left mouse button twice to highlight th..
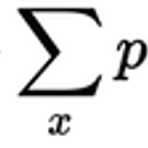
Cross entropy 에 대해서 명쾌하게 설명해주는 글! https://stackoverflow.com/a/41990932 What is cross-entropy? I know that there are a lot of explanations of what cross-entropy is, but I'm still confused. Is it only a method to describe the loss function? Can we use gradient descent algorithm to find the stackoverflow.com Key takeaways: 크로스 엔트로피는두 확률 분포의 차이를 구하기 위해서 사용된다. 딥러닝에서는 실제 데이터의 확률 분포와, 학습된 모델이 계산한 확률 분포..
Coursera - NLP in tensorflow 수업 takeaway 예를 들어서 classifier 를 만든다고 할 때, 그냥 Dense net 을 이용해서 만든다는 것은 결국 단어 순서 상관없이 bag of words 로 classifier 를 만드는 거다. 물론 이것도 충분히 잘 작동할 수 있다 - imdb 로 영화 리뷰 분류하는 것등. 그런데 tensorflow 기능 중에는 subword encoder 라고, 단어를 n-gram (n 이 fixed size 는 아님) 으로 tokenize 해주는 기능이 있다. 장점은 OOV 가 없다는 것이다 (새로운 단어도 다 더 작은 단위로 쪼개서 encode 하니까). 반면에, 이렇게 tokenize 된 text 를 보통처럼 embedding, poolin..
Subclass code example - build a model with tf.keras API https://gist.github.com/sthalles/190fcf1376fd0576ee3d6cd7f7c4b85d#file-subclassing_model-py subclassing_model.py GitHub Gist: instantly share code, notes, and snippets. gist.github.com Code details on https://towardsdatascience.com/everything-you-need-to-know-about-tensorflow-2-0-b0856960c074 Everything you need to know about TensorFlow 2.0..
BERT 를 이해하기 위해 읽은 글들 1. https://medium.com/dissecting-bert/dissecting-bert-part-1-d3c3d495cdb3 개괄적인 이해에 좋음. 인풋 행렬의 임베딩이 어떻게 되는지 실제 행렬 예시를 들어 보여주기 때문에 이해 하기 좋음. Dissecting BERT Part 1: The Encoder This is Part 1/2 of Understanding BERT written jointly by Miguel Romero and Francisco Ingham. If you already understand the Encoder… medium.com 2. http://jalammar.github.io/illustrated-transformer/ 내부 구..
- Total
- Today
- Yesterday
- language model
- Elmo
- Pre-trained LM
- word embedding
- nlp
- 벡터
- GPTZero
- Attention Mechanism
- 언어모델
- transformer
- neurone
- LM
- 뉴런
- Statistical Language Model
- weight vector
- Contextual Embedding
- neural network
- cs224n
- 뉴럴넷
- Bert
- Neural Language Model
- 워터마킹
일 | 월 | 화 | 수 | 목 | 금 | 토 |
---|---|---|---|---|---|---|
1 | 2 | 3 | 4 | |||
5 | 6 | 7 | 8 | 9 | 10 | 11 |
12 | 13 | 14 | 15 | 16 | 17 | 18 |
19 | 20 | 21 | 22 | 23 | 24 | 25 |
26 | 27 | 28 | 29 | 30 | 31 |